29 March 2020
Market insightsHave you got what it takes to create an integrated and seamless approach to marketing mix modelling? You'd be surprised how much you've got to gain.
In part 3 of our marketing mix modelling series, in partnership with Analytic Edge, we explain how emerging technologies and business practices have led to drastic improvements in the speed, precision and cost of our powerful prescriptive analytics engine.
Missed out on our last part of the series? Read part 2: Democratising marketing mix modelling
In Search of a Multi-channel View of Marketing Effectiveness
As digital ad spending has grown, tools like Multi-Touch Attribution (MTA) and customer analytics have become central to measuring marketing effectiveness. The granular, user-level view they provide makes a compelling case, allowing decision makers to interrogate both the ‘how’ and the ‘why’ of customer behavior. But emerging privacy concerns and new regulations have limited just how much of the total marketing spend these tools can cover. When marketers cannot effectively link behavior to specific personas or trackable IDs, their most compelling advantages can get lost.
Additionally, major ad platforms have moved towards a walled garden approach, restricting access to user-level data, and requiring marketers to use proprietary measurement tools and data. The net effect is that tools like MTA have become less strategic. They can no longer explain as much of the marketing budget as they once did. Brands that invest marketing dollars in acquisition, or on channels where they do not have full customer data, need to find other measurement options to give themselves a complete view.
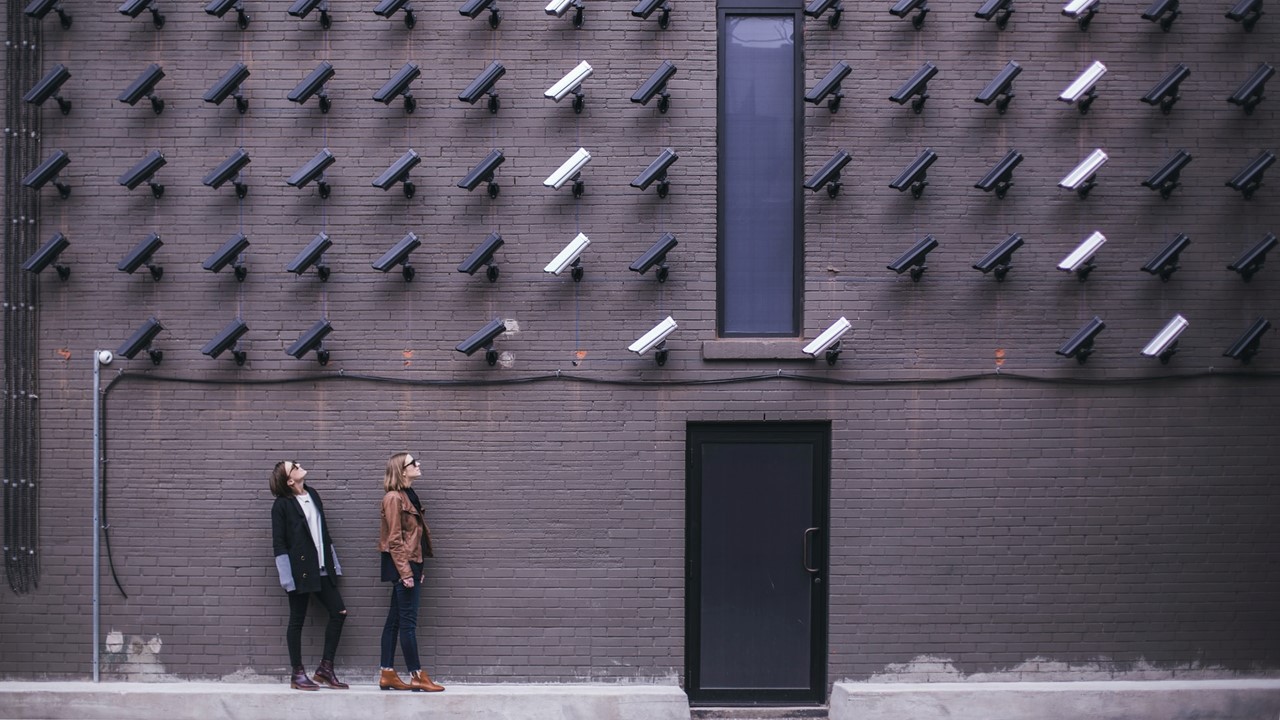
Marketing Mix Modeling for Strategic Perspective
For all these reasons Marketing Mix Modeling (MMM) is making a comeback. MMM has been used since at least the 90s, but the value it can provide has grown significantly. Gains in cloud computing, an explosion of marketing data and better BI and data science options have all expanded what MMM can offer. The benefits are largest when MMM can be delivered as part of an integrated platform, capable of delivering the ‘always on’ decision support marketers have come to expect from modern marketing-measurement tools.
Many firms would like to build an integrated MMM program in-house, the way they have with other data science and customer analytics work. If they already have a large data science team, then it seems like a natural extension. But MMM requires domain expertise to get started. And working with data science tools alone may not be enough to automate the process to the point where it can scale and reach its potential at a program level. Implementing an MMM program from the ground up is possible, but it takes time and can require significant upfront investment.
How to Get Started?
So how can firms add an MMM capability? What are the key factors that must be addressed when designing an MMM program? We offer this post to help companies assess their readiness for that journey, and to help them understand the key factors that need to be addressed before MMM can become a strategic marketing measurement asset.
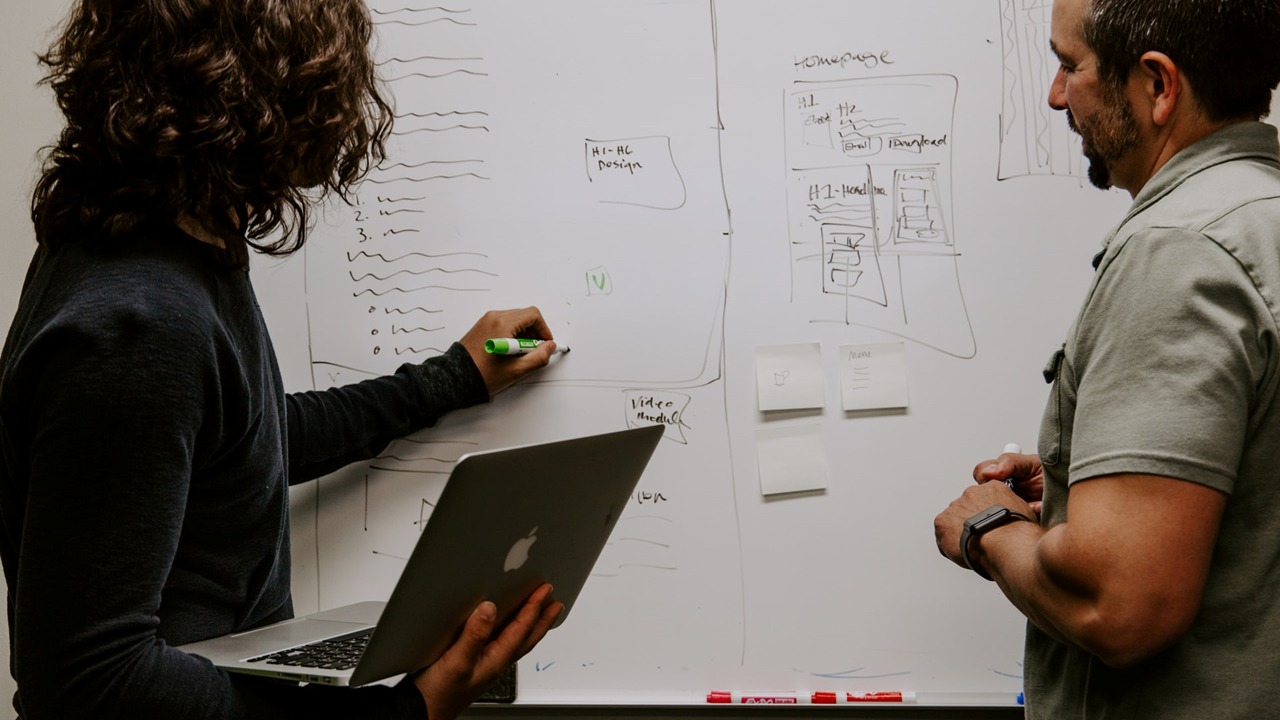
Data is the foundation
It goes without saying that you need sales and marketing data to analyze the impact of marketing on sales. But what are the key data sources and how should they be organized? What needs to be in place to get started? Companies have different business models with different KPIs and marketing approaches. But there are some common fundamentals:
- Sales & Revenue related KPIs
Different companies will focus on different metrics, but every company has a way to measure overall topline growth. Start by establishing access to this data at the same level you make your marketing investments. If you have channel or country specific marketing to measure, then you’ll want data at that level of granularity, or ideally lower (e.g. stores within a country or region).
- Marketing data
Start by focusing on the largest investments first. Set a goal of covering 60-80% of the budget and grow from there. Consolidating this data in one place is usually the main challenge. Start by looking in these areas:
- Digital
Everything from social campaigns to banner ads can be relevant. New players are emerging to help consolidate this data (e.g. Datorama, Funnel.io, Adverity). But it can also be sourced directly from the ad platforms themselves with the right technical expertise.
- Conventional media
TV from all sources (OTT, Linear, CTV) as well as radio can be important. Legacy sources like Nielsen and Kantar can help. But don’t overlook emerging sources like iSpot, Alphonso or InscapeTV. Cost can be an issue, but media agencies can sometimes help with topline access.
- Promotion and Trade Marketing
Look towards internal systems that track trade spending and co-marketing dollars. Retrieve this data at the same level as your Sales and Revenue KPIs or lower. Consider syndicated POS or price monitoring sources for a competitive view.
- Sponsorships, events, special programs
These investments can be difficult to measure, but there are always options. Start by focusing on reach/impressions for these programs if you can, then consider perception-based measures for key customer segments. At a minimum, track timing, spend and location if relevant.
You Need A Scalable Process
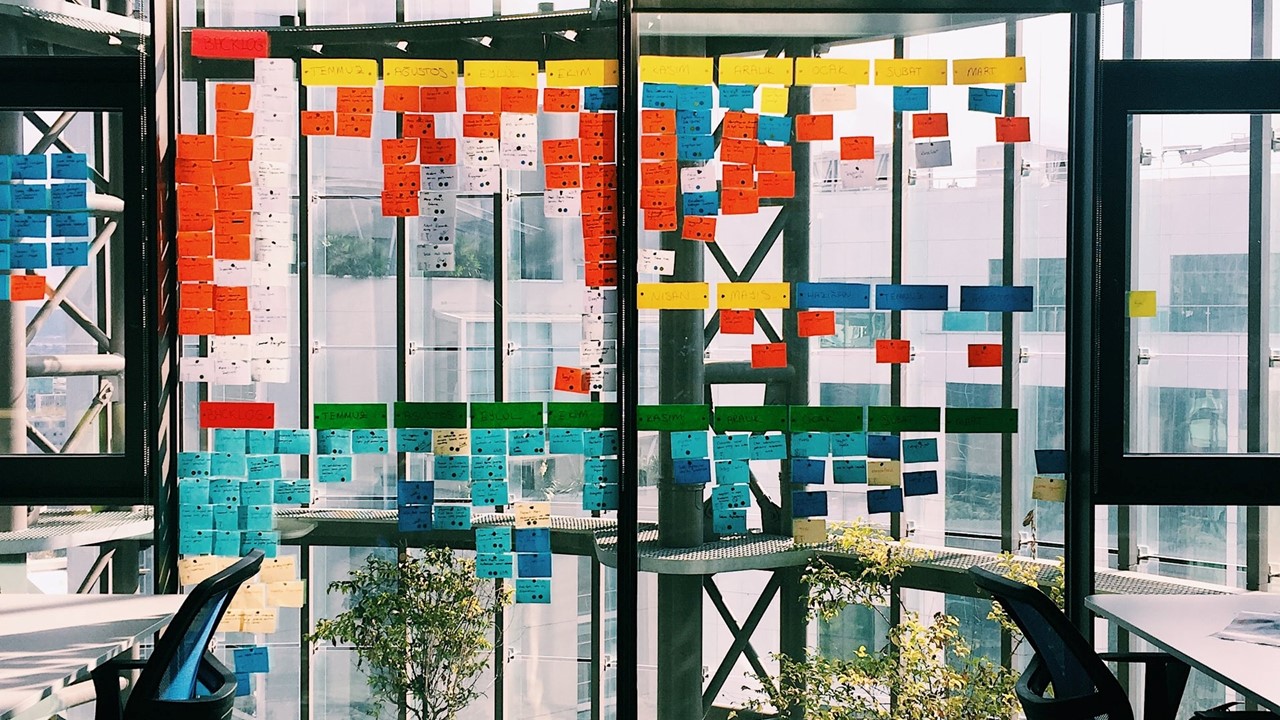
This is the step that stops most companies. The right data is ultimately available in most cases. But companies often lack the MMM-specific domain expertise required to build an MMM platform. Even when they have that expertise, they may lack integrated systems that can streamline the modeling workflow and automate the end-to-end analysis and reporting process. This includes both fundamental data review and QA tasks, as well as strategic marketing plan optimization and simulation exercises. Both are crucial to achieving the kind of scale, costs, and ‘always on’ guidance that marketers need, so they should both be incorporated. To achieve these goals, we focus on the following principles:
- Workflow automation
Automate the entire analysis cycle, removing custom coding from regular delivery wherever possible. Make model diagnostics and data review visual and intuitive. The goal is not to remove expertise or attention to detail. Instead, your goal is to help your team to cover more ground with less effort, and fewer mistakes.
- Data automation
Automate data ingestion and transformation for all required modeling inputs. They should be ready to add to your analysis without writing code or doing custom Extraction, Transformation & Loading (ETL) work, and come with the ability to make changes on the fly without digging into the back-end process.
- Transparency
With so much automation on deck it becomes even more important to have a transparent process. Make it easy for your experts to look under the hood, so they can validate the underlying code, adapt to changes, and evolve the process over time.
- Access & Collaboration
Make this entire process accessible from any location, ideally through a web front end that can accessed on your cloud. Build a system that supports all participants in the process, from the analysts reviewing and updating results to the marketers and planners who will rely on those outputs to make decisions. Make it a full end-to-end conversation.
- Organizational Fit & Familiarity
The right solution will also take advantage of the tech standards and tools already deployed at your company. It will allow you to leverage existing cloud, data science, and reporting assets, and support stakeholders with reporting and business intelligence (BI) options they find familiar.
- Cloud compatibility
Ensure the solution works in your cloud, whether its AWS, Azure, Google or something else. The components should be compatible with your environment and should work with your preferred tools to make maintenance and upgrades less of a risk.
- Support for Existing Reporting Tools
Ensure the solution can feed your preferred BI and visualization tools. The right tool will probably have embedded custom visualizations within the workflow, to keep the analysis fully integrated. But results should be easy to export to your existing BI and visualization tools and technologies.
Moving Forward – Demand Drivers as an Option
Every firm is different. We all come at the challenge of marketing measurement with a different set of priorities and different resources. But the points outlined above should help any company that needs a broader, more strategic view of their marketing investments to move towards that vision.
Analytic Edge’s Demand DriversTM is available to support this journey. Demand DriversTM is an end-to-end MMM solution that integrates the entire MMM workflow into a single platform, helping marketers achieve the strategic view they need, with ‘always on’ decision support, and no need to build their MMM system alone. As a cloud-based solution, it allows for easy implementation and integration with existing data sources and visualization tools, and it comes with training and technical support as part of the package. For more information, click here.
One Picture's Advanced analytics are delivered in partnership with Analytic Edge a leading global provider of cloud based always online dynamic modelling. Analytics Edge is a key supplier to Coca Cola, Mondelez, Unilever and Facebook.
Like this article?
If you'd like to hear more, subscribe to Frame or live chat to the team right here on this page.